Any time equipment is not operating during working hours, production suffers, and the company loses money. As a result, conflict often arises between operations and maintenance whenever a troubled asset is detected.
The maintenance department wants to repair the asset to prevent unscheduled downtime. Operations wants to keep it going as long as possible to meet production quotas. In an environment of tough deadlines and aggressive production goals, just identifying troubled asset isn’t enough.
Today’s maintenance and reliability professionals need to have answers to the questions of “How bad is it?” and “When should we act?” The new generation of cloud-based condition monitoring equipment puts the answers to those questions at the fingertips of operations and maintenance at a moment’s notice, no matter where they are.
Equipment Insight
Route-based monitoring might be adequate for noncritical assets with a low frequency of failures, but this type of monitoring only supplies an instantaneous reading; the asset may begin to degrade immediately afterward without any warning being given. The new generation of cloud-based condition monitoring systems provides a reliable and economical alternative.
When route-based monitoring identified a troubled asset in the plant of a major food processor, a third-party service vendor used online condition monitoring to maximize production while preventing catastrophic failure.
The 100,000-square-foot production floor housing the bottling line for the company’s signature barbecue sauce was served by a single rooftop blower. If the blower failed, production had to be shut down until repairs were made. Depending on the circumstances, the cost of downtime ranged from tens of thousands of dollars to as much as $1 million.
When route-based monitoring detected a defect in one of the blower’s bearings, operations wanted to continue production uninterrupted until the bearing was just about to fail.
The reliability expert, Ken Paterson of Koontz Wagoner, had to answer several key questions: How bad was the bearing? How long could production continue? Would it last for 24 hours or 24 days?
Paterson installed an online condition monitoring system on the blower (see Figure 1). He attached one sensor each to the inboard and outboard sides of the motor and the fan. The next steps were to set triggers and begin collecting data.
Figure 1. This online condition monitoring system features four sensors, each with a triaxial accelerometer and a temperature transducer. They are connected to the sensor node by up to 30 feet of cable. The sensor node features multiple mounting options and communicates via Wi-Fi or cellular network. When used with an optional encoder, the unit can also track speed.
Monitoring the Blower
Almost immediately after the unit was installed, Paterson was able to view the root-mean-square (RMS) data, the time waveform and the vibration frequency spectrum from each sensor on the fan and motor. From the beginning, the frequency spectrum showed a high peak at 1x fan shaft speed, as well as multiple harmonics (see Figure 2).
He set fast Fourier transform (FFT) bin alarms for 1x, 2x and 3x of the fan shaft speed. Meanwhile, production continued uninterrupted.
Figure 2. The vibration frequency spectrum of the fan outboard bearing showed high initial peaks at 1x fan shaft speed (red dot at left) and harmonics. This information was used to determine how to set alarms.
A week or so later, the magnitude of the RMS vibration for the outboard fan bearing began to rise, as the defect developed (see Figure 3). Paterson received alerts via email.
Figure 3. The RMS trend of horizontal, vertical and axial vibration of the fan outboard bearing for one month shows the emergence of the bearing defect.
Less than a month after the installation, further degradation to the bearing triggered RMS alarms and automatic email notifications. A review of the time waveform and vibration frequency spectrum revealed the presence of multiple harmonics of 1x fan shaft speed (see Figure 4).
Figure 4. The vibration frequency spectrum from the outboard fan bearing taken less than one month after the installation shows a 98 percent drop in the 1x peak (red dot at left), accompanied by sharp increases in the amplitude of the 2x and 3x harmonics. The spectrum also shows the emergence of additional harmonics, indicating looseness in the bearing.
The waterfall plot shows the development of the defect over the course of the month (see Figure 5).
Figure 5. Waterfall plot of frequency spectra highlights the emergence of harmonics as the bearing defect worsens. Data is displayed chronologically from back to front.
Figure 6. The time waveform shows the change in the bearing vibration.
After Paterson and the customer reviewed the data, they decided to delay repair until the end of the shift. The online condition monitor would alert them of any sudden changes in the status of the bearing before it became critical. The defect did progress considerably.
By the end of the day, the vibration was audible. At that point, the fan was shut down and repaired overnight. Between detection of the bearing defect and replacement of the unit, 53 days elapsed.
When the fan bearing was dissected, it showed signs of spinning in the housing. There was also evidence of a bearing-to-shaft fit issue. Visible scuff marks appeared on the outer and inner race of the bearing and inside the bearing housing (see Figure 7).
Figure 7. Dissection of the bearing revealed visible damage on the inner race (left) and outer race (right).
Getting Started with Online Condition Monitoring
The example above shows the value of the technology. Although this was a case of reactive maintenance, online condition monitoring is more frequently used to support predictive maintenance. The same techniques can be used to characterize the performance of a healthy asset and make it possible to detect any problems well in advance.
At that point, the same questions (How bad is the problem? How soon does maintenance need to act to repair it?) can be definitively answered.
The best way to explore the benefits of online condition monitoring is to launch a pilot program. This will both demonstrate value and provide a small-scale testbed to learn best practices.
Start with a thorough inventory of all assets likely to fail. Vulnerable assets typically include motors, gearboxes, actuators, couplings, clutches and brakes, etc. Classify them by the likelihood of failure and rank them by the impact that failure has to operations.
The combination of this information makes it possible to rank the priority of continuous monitoring (see the table below). Use this information to identify a potential asset or class of assets for a predictive maintenance pilot program.
Once you have identified an asset or asset class, the next step is determining the cost of downtime for that asset. Go beyond loss of production and cost of repair. Include factors like cost of work in process, decreased production on other machines, cost of idle labor and penalties for late delivery. Use this information to sell the value of predictive maintenance within your organization.
Continuous online condition monitoring solves the conflict between operations and maintenance. The visibility it provides makes it possible to maximize production, optimize equipment lifetime, and, of course, prevent unscheduled downtime.
In the example above, online condition monitoring enabled the asset owner to run the equipment for an additional 52 days after the defect was first identified, and to do so with confidence. When the maintenance department did eventually make the repair, they did so outside of normal working hours so that it did not impact operations.
How bad was the defect? When did maintenance need to act? Continuous online condition monitoring had the answer.
This article was previously published in the Reliable Plant 2019 Conference Proceedings.
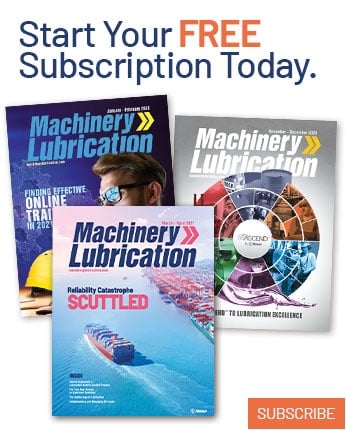