The life and reliability of greased machinery depend on proper bearing lubrication. Unfortunately, inadequate grease lubrication is a very common failure mode. With greased bearings, it is difficult to determine lubricant state along with knowing when to grease, how to grease and when to stop greasing. Fortunately, predictive technologies and work processes are being successfully applied to monitor and optimize the lubrication condition.
Improper lubrication creates energy that manifests itself as mechanical stress energy and thermal energy (heat). By analyzing this energy using predictive maintenance (PdM) technologies, much can be learned about the bearing lubrication condition. This article discusses and compares the use of ultrasonic analysis, conventional vibration analysis and advanced vibration signal processing techniques as means toward determining lubrication state and achieving optimum lubrication.
Specifically, this article will look at:
- the characteristics and progression of lubrication stress,
-
an overview and comparision of
- ultrasonic analysis
- conventional vibration analysis and
- advanced vibration analysis
- the strengths and weakness of each monitoring method,
- the characteristics of various lubricant states obtained in a controlled test environment, and
- equipment lubrication case studies.
Traditional greasing programs use time-based preventive maintenance (PM). This method can result in over- or under-greasing, depending on the recommended periodicity of greasing, operating conditions and run time of the machinery. One plant surveyed stated that 90 percent of all motor failures were grease related – both over- and under-greasing. This particular plant was using motor manufacturer recommendations as the foundation of their greasing program.
An alternative greasing program uses PdM processes and technologies. Using PdM, greasing activities are based on the condition of the grease. Several PdM technologies for grease monitoring are available. Vibration tends to be the most common tool used. However, most commonly used vibration techniques do not detect early lubrication faults. Infrared is also an option, although it is not preferred since initial lubrication starvation has little impact on temperature. Some sources maintain grease sampling and analysis is the best method. However, in most circumstances, this is not practical. Many bearings do not have an access port to the grease and those that do present their own problems. The grease samples taken most likely do not represent the true condition of the grease inside the bearing and may also contain particulate and contamination picked up during the sampling. Ultrasonic analysis and advanced vibration signal processing are viable methods of monitoring grease condition since they measure the stress energy caused by friction between the rolling and sliding elements in the bearings.
Section 1: The Characteristics and Progression of Lubrication Stress
In rolling element bearings, lubrication sound is created by friction-induced stress waves from the interaction of the roller to the race and the roller to the cage. Good roller-to-race interaction is rolling with elastohydrodynamic lubrication. Good roller-to-cage interaction is sliding with hydrodynamic lubrication. Assuming a RMS surface roughness of 0.3 micron, Figure 1 shows that the minimum lubrication film thickness for the roller-to-race is 1 micron, or approximately 1/75 the thickness of a human hair. The minimum lubrication film thickness for the roller-to-cage is 10 microns. As lubrication starvation occurs, the film thickness will decrease, resulting in a greater coefficient of friction. The greater coefficient of friction creates additional energy in the form of heat and sound.
The lube sound characteristics for under-lubricated bearings are:
- high frequency energy,
- broadband energy, and
- primarily random and non-periodic noise.
Figure 2 shows the vibration frequency domain of an under-lubricated bearing. Note the high-frequency energy hump in the 20 to 32 kilohertz (KHz) range.
Figure 3 shows the vibration frequency and time domain for a gearbox running with an incorrectly installed oil pump. Note the high frequency in the 10 KHz to 20 KHz range. This energy probably continues to higher frequency range but shows a drop-off due to the accelerometer range and mounting conditions. The time domain shows a raised noise floor with random high spikes.
As the lubrication in a bearing degrades, the high-frequency sound characteristics change. A properly lubricated bearing creates random noise with a low noise flow. As the lubricant degrades, the noise floor begins to elevate (Figure 4, Chart 1). Listening to a heterodyned 30 KHz signal, the sound is like a waterfall or rushing river. As the lube degrades, spiking begins as the noise floor elevates which sounds like a mild popping sound (Figure 4, Chart 2). Severe lubrication faults cause more frequent high-amplitude spikes, which sound like bacon sizzling. In Chart 2, as grease was added, the noise floor immediately lowered and the spiking disappeared.
Do not expect bearing to display the same sound characteristics. The amplitudes, sound patterns and frequencies of the stress energy are influenced by factors such as:
- Speed
- Bearing geometry
- Roller design and quality
- Load
- Cage design
- Type of lubricant
The following summarizes key points related to the characteristics of lubrication sound/stress.
- As lubrication condition degrades, stress energy increases.
- Lubrication stress energy is broadband, high frequency (about 8 KHz and higher) and random.
-
Other sources of high-frequency stress energy exist, such as:
- Bearing/gear defects, electrical – periodic
- Rubs, fluid turbulence – random
• To evaluate lube condition, high-frequency diagnostic tools that can measure and differentiate random from periodic noise are required.
Section 2: Overview and Comparison of Ultrasonic Analysis, Conventional Vibration Analysis, and Advanced Vibration Analysis
When monitoring for lubrication faults, sensor selection is critical. Sensors are not the same, so the characteristics of a sensor must be understood. Figure 5 shows the sensor performance of several different diagnostic technologies. The red broad-band sensor would be a poor choice because the high-frequency sounds would be drowned out by the low-frequency noise. The blue narrow-band sensor in the bearing fault area would completely miss any lubricant stress noise. The black broad-band sensor represents a typical accelerometer. It may have a response in the lubrication range, but mounting techniques are critical (see Figure 6). The blue narrow-band sensor in the lubrication area has good sensitivity and completely isolates itself from lower-frequency noise.
Figure 6 shows that when using an accelerometer to monitor lubrication stress, either a flat magnet on a clean machined surface or a permanently mounted accelerometer must be used to get the required frequency response.
Five technologies to identify and monitor lubrication faults are:
- Infrared thermography
- Listening using an accelerometer
- Ultrasonic
- Conventional vibration analysis
- Vibration analysis with advanced signal processing using PeakVue and Autocorrelation
The next few paragraphs will briefly introduce each of these technologies. This will be followed by side-by-side comparison test results for the latter three.
Infrared thermography is good for detecting possible late-stage lubrication problems. It is not good for catching lubrication problems early. Also, additional technologies will be required to confirm the source of the abnormal temperature signature.
Some vibration analyzers have a headphone output to listen to the accelerometer output (Figure 7). This can be used to listen to the noise within the bearing. A high-pass filter on the sound output is very useful to filter out the low-frequency noise so that the lubrication noise can be heard. This is a quick qualitative method that provides good signal pattern information. It can also indicate a drop in volume, while greasing can indicate that the grease has reached the rollers.
Ultrasonic monitoring provides both listening capability and quantitative measurements (Figure 8). The ultrasonic sound is heterodyned into our listening range, and specific sound parameters are measured. The ultrasonic device should isolate the higher frequencies and filter out all lower-frequency noise. If the ultrasonic device uses a narrow-band sensor or resonant sensor, mounting is much easier than with an accelerometer. Generally, just placing the probe on a clean surface provides a good signal. Paint and other obstructions will lower the signal amplitude.
Conventional vibration analysis analyzes macro vibration – the motion of a body. It focuses on the lower-frequency events. For an 1,800 rpm motor, a typical maximum frequency would be 60 times running speed, or 1,800 hertz (Hz). Figure 9 shows a typical frequency domain plot.
The CSI PeakVue measurement is an advanced signal-processing method. It performs micro vibration analysis, which measures the microscopic movement (stress waves) within a body. It analyzes the energy above the conventional analysis range. PeakVue is not an averaging process, so it captures the true peak amplitude values from the high-frequency information.
To create the PeakVue time waveform, the accelerometer signal is routed through a high-pass filter to cut out all low-frequency information. The signal is sampled at more than 100,000 times per second. This digital data stream is broken into data blocks which are a function of the desired Fmax. The only value saved for each data block is the true peak “G” amplitude value. These true peak G levels create the PeakVue time waveform. This PeakVue time waveform is then processed to create a PeakVue frequency domain spectrum (Figure 10).
PeakVue provides a window into the area where lubrication data is located. The nature of lubrication faults make the PeakVue time domain data noisy and difficult to interpret. To assist with this, another advanced signal-processing technique, CSI Autocorrelation, can be applied. Autocorrelation is an averaging process to remove random activity from the time domain. The resultant correlation factor (Figure 11) is proportional to the amount of periodic energy. If at any point on the correlated waveform the correlation factor is small, then almost all of the energy at that point is random.
Figure 12 shows a test motor. To compare ultrasonic, conventional vibration analysis and advanced vibration analysis, the top inboard bearing was monitoring both with and without lubricant. The next few paragraphs will compare the results from the three technologies. For the vibration measurements, a mounting pad was glued to a machine flat on the bearing housing. A flat magnet with accelerometer was attached to the pad. A CSI SonicScan was the ultrasonic test device. It measured both lower-frequency energy (4 KHz) and ultrasonic energy (30 KHz).
Figure 13 compares the time domain data for the three technologies. The conventional vibration data shows almost no difference between lubrication states. PeakVue clearly measures the stress energy caused by the lubrication fault. The SonicScan data was consistent. The lower-frequency energy measurement showed some but little change. The ultrasonic data showed significant change in both the average reading and peak amplitude reading.
Figure 14 shows a comparison of the frequency domains. With conventional vibration, a slight change in periodic activity is noticed. This is likely because the existing bearing mechanical defects are being amplified a little without lubricant. The PeakVue spectrum shows a dramatic increase in stress energy.
The PeakVue time domain for the no-lubricant condition is messy and hard to interpret. When the Autocorrelation is performed (Figure 15), it is immediately apparent that almost all of the stress energy is random, which is a primary characteristic of lubrication faults.
Table 1 provides a summary comparison of the discussed technologies. The left side of the table shows technology features that make testing accurate and easy. Accelerometer listening and conventional vibration analysis will not provide good results. Headphones should be used when performing advanced vibration analysis to add a very important qualitative attribute. Advanced vibration analysis provides the best information for determining lubrication condition but requires additional vibration analysis training and special sensor mounting. Ultrasonics provides a good indication of lubrication condition (without access to detailed signature analysis tools) and are easy to use but also require some specialized training.
Section 3: Characteristics of Various Lubricant States Obtained in a Controlled Test Environment
To better understand and verify theoretical assumption, a lubrication test stand was built for controlled lubrication testing. Figure 16 shows the test stand that was used to collect the controlled grease lubrication testing data.
A motor drives a control, idler and test bearing. Grease can be introduced into each bearing through its outer race using a zerk fitting on the bearing housing. The idler bearing can be variably loaded to 1,000 pounds. The bearings tested were sealed SKF single-row ball bearings with nine balls. The bearings have an ABEC 1 rating and were driven at 1,780 rpm. For each lubrication condition, peak and average decibel (dB) levels were measured at three frequency ranges. The ranges were 30 kHz (lubrication range), 4 kHz (impact range) and 40 kHz (traditional ultrasonic range). The peak dB level records the highest signal amplitude, such as in a spike, during a data collection period. The average dB level records the average of all the signal peaks during a data collection period and is least affected by spikes. The sound parameters were collected using a Model 7100 SonicScan analyzer using a magnet mount sensor. A magnet mount sensor allowed for hands-free operation and greater repeatability. The temperature was monitored on the bearing housing using a CSI Model 515 spot radiometer with laser pointer.
First, to evaluate the effects on sound of over-lubrication, a new bearing running at steady state under load was slowly greased until grease squeezed through the seals (see Figure 17).
Figure 18 shows no significant increase in sound up to 12 minutes after over-greasing. Over-greasing did create a 7 percent rise in the temperature on the outside of the bearing housing. It should be noted that the rate of grease oxidation and deterioration will increase with increasing temperature.
Second, to evaluate the effects of under-lubrication, a new bearing with seals removed was brought to steady-state temperature under full load. The bearing was stopped long enough to wipe grease off of one side of the bearing and then retested. The bearing was then washed in solvent to remove all grease (see Figure 19) and then retested. When the bearing with no grease was approaching total failure, it was progressively regreased and monitored for 30 minutes until it reached steady state.
Figure 20 shows results from lubricant starvation and then re-lubrication. The dB levels increase with deteriorating lube conditions and they decrease with improving lubrication conditions. The temperature remains constant except when lubricant is absent. While temperature is a good indicator of total lube starvation, it was not a good indicator of partial lube starvation.
Seen from Figure 20, the initial re-lubrication made a significant improvement in sound level and temperature even though only one pump, or 0.04 ounces of grease, was introduced. Figure 21 shows the sound waveform (heterodyned 30 kHz audio output from SonicScan) immediately prior to and after the first pump of grease. A very distinct drop in level occurs when the grease hits the rollers. Additional greasing resulted in a minor sound level decrease and a negligible effect on temperature. Permanent damage likely occurred to the bearing since the sound levels did not return to their original levels.
The results of the sound monitoring in a controlled environment as they apply to lubrication monitoring can be summarized as follows:
- Sound analysis is a poor technology for monitoring over-lubrication since over-lubrication does not significantly affect the sound parameters,
- Temperature analysis is a good technology for monitoring over-lubrication since it does significantly increase with over-greasing; however, in the field, be aware of process and environmental effects on the temperature,
- Sound analysis is a good technology for monitoring normal and under-lubricated bearings since the sound levels increase with deteriorating conditions and decrease with improving conditions,
- Lubrication problems that are not corrected in early stages will result in permanent mechanical damage, and
- Listening during greasing for a decrease in the sound level is a good indicator of grease entering the bearing.
Section 4: Equipment Lubrication Case Studies
Lubrication ultrasonic analysis was performed on the following operating industrial equipment: food processing conveyor bearings, 75-horsepower General Electric motors, and 450 HP Baldor motor. The conveyor testing occurred during a single day, while the motor testing occurred over a seven-month period. The testing confirmed and validated the characteristics of lubrication sound and the findings from the controlled environment testing. The instrument used to analyze the sound was a CSI Model 7100 SonicScan with a multi-frequency contact probe (see Figure 22) instead of a magnet mount sensor. Measurements were taken on the top of the zerk fitting. In general, contact probe measurements are not as repeatable as magnet mount sensor because of variable contact angles and pressures. The advantage of the contact probe is that it can easily fit into tight places and can be used on zerk fittings.
Food Processing Conveyor Bearings
The survey included 13 bearings from a conveyor in a food processing plant. Most of the bearings were pressure-washed frequently with water and greased daily. Bearing No. 11 was elevated above floor level, in a difficult-to-reach position, and apparently not greased regularly.
Each bearing was tested three times before greasing and three times after greasing. Of all the bearings that were greased daily, the additional greasing had little or no effect on the bearing sound. Greasing significantly affected bearing No. 11. Figure 23 shows a summary of the readings taken at both the 4 kHz and 30 kHz frequency ranges. It also shows the peak (PH) and average (AV) readings at each frequency.
For each set of columns, the first column shows the mean dB reading of all 13 bearings. The second column shows the dB reading of bearing No. 11 before greasing. The third column shows the dB reading of bearing No. 11 after greasing. In both the 4 kHz peak and 4 kHz average readings, bearing No. 11 was slighter higher than mean before greasing and slightly lower than mean after greasing. The 30 kHz peak reading shows a significantly elevated dB before greasing and a significant dB drop after greasing. The greatest change occurred with the 30 kHz average reading with the average reading dropping from the upper 20s to almost zero. In this test, the 30 kHz average reading was the most sensitive parameter for measuring lubrication condition. It should also be noted that permanent mechanical damage was unlikely since the 4 kHz peak (mechanical impact) reading dropped to below mean and the 30 kHz average (lubrication) reading dropped to almost zero.
Headphones were used with the SonicScan to provide additional qualitative information. Unfortunately, in this environment, a lot of noise existed in all of the measurements. Metal cans were clattering on the conveyor, belts were sliding and the background was loud. For these or other reasons, the headphones did not provide useful information in this study.
75 HP General Electric Motors
Several 75 HP General Electric motors were monitored. The motors run at 1,780 rpm and drive blowers. Each bearing has a single shield toward the rotor. The grease cavity has a zerk fitting at 2 o’clock and an unused grease vent plug at 10 o’clock. The motors are greased every 3,500 hours with 12 grease gun pumps. Currently, the maintenance group has no idea of the lubricant condition before or after greasing.
Figure 24 plots the lubrication sound levels according to the number of hours on the motor since greasing. Notice that the inboard bearing dB level dropped to almost zero after greasing and remained constant through 766 hours. The outboard bearing performed similarly except that the dB level began to increase after 358 hours. This could be caused by a lot of factors, such as possibly only a small amount of the grease that entered the cavity actually made to it the bearing.
Figure 25 shows a similar plot for a second 75 HP General Electric motor. The 30 kHz sound level performed as expected. The 4 kHz dB level seemed to not be affected by greasing. The 4 kHz dB levels very likely can be affected by noise from other machinery and processes since sonic sound travels through material much better and attenuates much slower than ultrasonic signals. The 40 kHz signal did not perform as expected. In general, the 40 kHz signal is less reliable since the amount of lubrication energy created at 40 kHz is less than what is created at 30 kHz.
450 HP Baldor Motor
Several 450 HP Baldor Super E motors were monitored. The motors run at 1,780 rpm and drive large blowers. Each bearing is neither shielded nor sealed. The grease cavity has a zerk fitting at 12 o’clock and an unused grease vent plug at 6 o’clock. Access to the grease vents is restricted because of the design of the motor mount. The motors are greased every 3,500 hours with 24 grease gun pumps. Currently, the maintenance group has no idea of the lubricant condition before or after greasing.
Figure 26 plots the lubrication sound levels according to the number of hours on the motor since greasing. At 3,411 hours, the bearing was greased – first four pumps and then an additional four pumps. With the 30 kHz readings, the sound levels dropped significantly, while they increased slightly with additional grease. Because of this increase, greasing was stopped. Only after 552 hours, the sound level returned to its previous level, that is most likely a state of adequate lubrication. Like with the GE motors, the 4 kHz sound was not affected by the greasing.
Figure 27 charts another Baldor motor bearing. At 30 kHz, this bearing has a steady sound level until 3,278 hours. After greasing, the sound level dropped back close to the previous level.
The results of the sound monitoring in an industrial environment as they apply to lubrication monitoring can be summarized as follows:
- In all of the GE and Baldor bearings, the 30 kHz average reading provided the most predictable readings. The 40 kHz readings generally followed the 30 kHz readings but were less consistent.
- Greasing did not affect the 4 kHz readings. The 4 kHz reading can be very useful in analyzing the bearing’s mechanical condition or as a confirmation technique for conventional vibration analysis.
- Trying to determine lubrication condition by listening alone is ineffective since the lubrication sound is primarily white noise. Quantifiable and repeatable analysis is required to accurately assess lubrication condition. The qualitative information from listening is very useful for general listening and checking for other problems. It can also be useful during lubrication to determine when grease enters the bearings.
- For all of the industrial bearings tested, optimum or baseline lubrication levels (30 kHz frequency and average sound parameter) were around 10 dB or lower. The normal operating lubrication levels were around 10 to 20 dB. For the test stands bearing and conveyor bearing, the critical level before permanent damage occurred was around 30 dB. This would imply that the greasing level should occur between 20 and 30 dB. These estimates are very general. More exact levels should be determined by type of bearing and application. This could be accomplished through testing and long-term trending.
- All the bearing testing did not exhibit a standard lubrication cycle except that increasing hours eventually resulted in increasing sound levels and that re-lubrication resulted in decreasing sound levels except for the case of already over-lubricated bearings. Specific lubrication cycles for specific bearings could be understood through long-term trending. This would provide much information on the real-time lubrication condition and projected performance.
- By trending the sound levels along with the lubrication intervals and grease amounts, the grease interval and volume can be optimized. The most favorable temperatures will be obtained when the bearing is supplied with the minimum quantity of lubricant to provide proper lubrication. Therefore, as a general rule, less grease is better than more grease. The trending will reveal the cause and effect of lubrication quantity on lubrication condition and interval. Lubrication monitoring with vibration was performed on a pump, scrubber fan and exhaust blower.3
Figure 28 shows the conventional vibration data for a pump. This data shows no indication of a lubrication fault. The PeakVue data for the same pump shows high G levels and random patterns which could indicate a lubrication fault (Figure 29). The autocorrelation of the PeakVue waveform confirms that the energy is almost all random (Figure 30). The pump locked up one month later due to lack of lubricant.
The next vibration case history is for a scrubber fan. The PeakVue data on April 19 (Figure 31) was below the alert level. On May 29, the PeakVue data (Figure 32) was in alarm with a 500 percent increase in G amplitude. The autocorrelation of the May 29 data (Figure 33) revealed no periodicity. This random high-frequency energy is a strong indicator of a lubrication fault. Figure 34 shows a comparison of the PeakVue time waveforms. Figure 35 shows a comparison of the conventional time waveform data. Note that no increase occurred with the conventional data (Fmax of 3 KHz). The source of the lubrication fault was a faulty oiler bowl. As soon as the oiler bowl was fixed, the PeakVue data returned to normal levels (Figure 36).
The third vibration equipment case study is for an exhaust blower. A new type of grease with limestone and graphite additives was used. Figure 37 shows the frequency domain before and after the new grease was added. The new grease caused significant high-frequency energy – 8 KHz and higher. Figure 38 shows the PeakVue time domain comparison. Notice the large increase in friction energy because of the different lubricant.
These three equipment case studies show that advanced vibration analysis using PeakVue and autocorrelation are effective techniques to identify and monitor lubrication condition. They also show that conventional vibration analysis is not as effective.
Conclusion
To achieve optimum lubrication, it is important to be able to determine:
- the lubrication condition at any time;
- the conditions when re-lubrication is necessary;
- the volume of grease required for re-greasing.
As seen from this article, multiple predictive technologies can provide immediate information about the lubrication condition while the equipment is operating. Each of these technologies has strengths and limitations. For effective lubrication monitoring, each of the technology’s strengths and weaknesses must be understood along with the physical characteristics of lubrication energy.
Lubrication analysis using advanced vibration analysis should be performed on all critical machines, especially if the equipment is already being monitored for bearing defects. If equipment is critical enough to warrant vibration analysis, then the lubrication can be monitored as a part of that process. Many companies do not monitor lubrication because of the cost to prepare a proper mounting surface for the accelerometer. This cost is insignificant when compared to the cost of a lubrication fault in terms of lost production, lost equipment life and higher maintenance costs.
When lubrication analysis is combined with good trending and monitoring, greasing intervals and amounts can be optimized. All of this results in knowing the equipment better and being better able to maintain it, extend its life, and predict its performance.
For more information on this subject, visit www.emersonprocess.com.
References
1 Chart based on information from “Improving the Reliability of Machines by Understanding the Failure of Their Moving Parts,” Master Series Course taught at CSI by M. Neale and D. Summers-Smith, October 1997.
2 “Machinery Surveillance Employing Sonic/Ultrasonic Sensors” by J. C. Robinson, J. B. Van Voorhis, K. R. Piety, and W. King, Reliability Week 1999.
3 “Monitoring Lubrication Using a Multi-Frequency Sonic/Ultrasonic Sensor” by Ray Garvey, Computational Systems Inc.
3 Three case studies from “Advanced Vibration and Autocorrelation Analysis for Improved Diagnostics”; James Robinson, Bob Cook, James Crow; Emerson Global Users Exchange, 2006
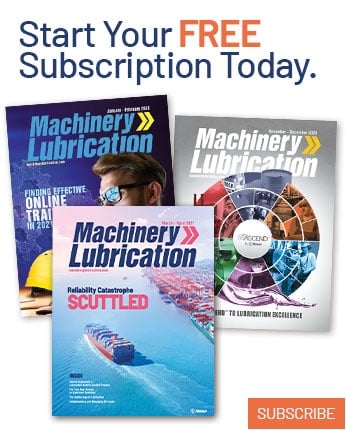