Unplanned downtime is one of the major challenges facing factory and building operators worldwide.
Industry data shows that, on average, a factory will experience 144 downtimes per year, and the average annual cost of these unplanned standstills for a factory is $2.3 million. In certain industries, such as automotive, these costs are even higher, with losses of tens of thousands of dollars accumulating for each minute of unplanned downtime.
But this isn’t the only challenge facing facility productivity. Almost one-quarter of the entire United States manufacturing workforce is over the age of 55, and by 2030, there will be up to 2.1 million unfilled jobs due to the growing skills gap. In a survey conducted by the Associated General Contractors of America, 32% of billion-dollar manufacturers estimate they will lose over $100 million over the next five years with the retiring of the Baby Boomer generation, and 74% of firms predict a shortfall of qualified, skilled trade workers.
On several fronts, plant managers are being asked to do more with less, making ongoing maintenance optimization a constant challenge.
The Core Maintenance Strategies
There are four core maintenance strategies generally practiced:
The first one is Reactive Maintenance, also known as “Fix it when it fails” or “Run to failure.” The maintenance and production downtime costs for this strategy are very high because the asset is run to failure. With this strategy, a facility must always keep spares available, increasing both the inventory and labor costs to repair the equipment. The main advantage of reactive maintenance is it reduces the cost of performing condition-based or usage-based maintenance. But eventually, the asset will fail. Therefore, this strategy is most effective with non-critical machines that are inexpensive and quick to repair.
The second strategy is Preventative Maintenance, also known as “Fix it before it’s due to fail” or “Interval-based monitoring.” This approach is valuable for assets with age-related failure modes where no cost-effective condition-based techniques can be applied. The primary mistake made when implementing preventative maintenance is it’s often applied too broadly, even on assets without age-related failure modes. This can increase downtime and result in excessive routine maintenance expenses. Every unnecessary maintenance action performed on a machine is an opportunity for new issues to be introduced.
The third type of maintenance strategy is Proactive Maintenance, or “Maintain it so it keeps running.” The concept is to do everything possible to reduce the likelihood of failure, including operating the equipment properly and conducting proactive maintenance tasks, such as cleaning and properly lubricating. The advantage of this strategy is it eliminates many of the root causes of equipment failure and extends the life of the equipment. The disadvantage is that it takes the support of management to schedule the time needed to perform these tasks.
The final maintenance strategy is Predictive Maintenance, also known as “condition-based monitoring of assets.” Predictive maintenance involves testing the condition of the equipment to determine if a failure will occur in the foreseeable future so that the components in question can be repaired or replaced at the most convenient time. While monitoring the machines alone does not stop components from failing, plant managers can extend the life of the asset at a minimal cost by more accurately predicting specific potential failures and replacing the failing components just before they are due to fail.
The advantages of predictive maintenance are reduced downtime, lower repair and spare inventory costs and the ability to avoid secondary damage. It is most valuable for situations where there are critical assets that have a high cost of downtime, assets with a history of failure or hard-to-reach assets where regular checks are difficult or dangerous.
To successfully implement this strategy, equipment should be fitted with sensors and connected to the Industrial Internet of Things (IIoT). Doing so will enhance the overall effectiveness of predictive maintenance techniques.
Industrial Internet of Things
An interconnected network of devices and machines, connected through the internet, that collects and communicates data directly to the maintenance team in real-time.Source: reliableplant.com
Connected Devices and IIoT Gaining Momentum
Predictive maintenance and the IIoT are tools that have been gaining momentum in the industry for years and have finally reached a point of maturity where solutions are easily accessible. This is crucial because, in a factory setting, a production line is only as resilient as its least reliable asset.
Looking at the industry holistically, the predictive maintenance market stood at $5.6 billion at the end of 2020, representing a 17% increase year over year. While impressive, these numbers are just the beginning. Survey data found that less than 40% of companies have invested in predictive maintenance, but within the next three years, more than 75% expect to have projects in motion.
As the share of assets being monitored by real-time smart sensors is set to grow, plant managers can use that data to rethink how these assets are being maintained on an ongoing basis.
Connected Assets Drive Condition-Based and Predictive Maintenance Approaches
The growth of IIoT-based solutions is unleashing a paradigm shift in machine health monitoring, allowing companies to gently shift away from manual walkarounds and legacy preventative maintenance schedules and toward condition-based and predictive asset monitoring approaches.
Condition-based systems are built around a sensor, such as vibration, pressure or temperature, connected to a monitoring platform via either a wired or wireless connection. Based on the machinery and processes in place, an alert value is set, which represents an error state or potential machine health issue. If the alarm is triggered, technicians are alerted, and the team works to identify the issue and replace the machine or part.
One example of this is the concept of using temperature sensors to monitor high-resistance joints in a motor where loose or corroded electrical connections can generate current arcing, a rise in temperature and a potential fire. When the temperature reading reaches a critical value, signifying a motor may be nearing a failure point, the maintenance team is alerted and can appropriately schedule corrective action.
Predictive maintenance takes the principles of condition-based maintenance and pushes them further, using machine learning and artificial intelligence (AI) to identify issues earlier. Rather than waiting for issues to reach a critical alert value, the system looks for anomalies in various sensing modalities and uses those values in combination with machine learning and AI algorithms to predict upcoming machine failures and identify the root causes of potential issues.
This dynamic is often represented using the PF curve (Potential Failure, Functional Failure), where the system can interpret different sensor readings and make judgments about the remaining useful life (RUL) of the asset. This type of evaluation can allow operators to determine the amount of time they have to make repairs and identify the optimal time to take corrective action, creating a minimum amount of disruption to overall operations. Because of these smart systems, facilities can begin conducting their own in-depth investigations, such as vibration monitoring, without hiring an outside expert.
Vibration monitoring specifically can create a strong return on investment for a wide variety of assets, as its principles can be applied widely to motors, pumps and other rotary equipment. Over 50% of motor failures are due to premature bearing failures. In addition, many of the rotating machine faults that can be diagnosed with vibration condition monitoring, such as unbalance, misalignment and mechanical looseness, directly impact the motor’s bearings.
Vibration analysis also ties directly to the ISO 20816 standard, which establishes how companies should evaluate machine vibration magnitude and shifts to help ensure reliable equipment operation. In most cases, vibration begins earlier on the PF curve than audible noise or temperature variations that are detected during a standard operator walkaround.
By using vibration analysis in combination with other sensor types, operators can identify potential machine health issues earlier in the process and approach corrective action on those assets with a long-term view. Repairs do not need to take place immediately but can be scheduled for the optimal time within the expected useful life of the asset.
How Plant Managers Can Avoid Pitfalls in Machine Health and Monitoring Programs
The first step in establishing a predictive maintenance or machine health monitoring program is to create an internal pilot strategy to identify the best assets for this type of platform.
Factors may include:
- Assets with high risk and/or history of failure: Some assets tend to fail more than others.
- Impact of potential downtime: Downtime with certain assets is more damaging than others and often goes beyond just the loss of production. When looking at the standard flow of work, understand whether an asset failure could lead to delivery failures, fines or strain on other production lines.
- Potential duration of downtime: Identify the lead time for replacement parts on each asset and how long the repair will take.
Once a company’s machine assets are viewed through these lenses, the highest-risk assets should rise to the top.
In choosing an IIoT system, factors to consider include:
- Ease of installation and scalability: Sensors should be easy to install and configure to minimize any downtime with system startup. With the advancement of wireless technology, the infrastructure required for them should be appropriate and limited.
- Relevant and accurate sensing modalities: Plant managers and integrators need to understand what sensors will deliver the correct data about the health of an asset.
- Intuitive data analysis tools: Systems should be designed to be managed by most members of the maintenance team and not be so complicated as to require the hiring of outside experts. While it is helpful to monitor vibration readings on their own, AI and machine learning algorithms may catch an anomaly that even experts can overlook.
- Costs and Return on Investment (ROI): Machine health monitoring systems should offer a compelling ROI in months, driven by a reduction in unnecessary maintenance actions, unplanned downtime and walkaround costs.
Digital Transformation for Smart Factories and Buildings
As these connected solutions continue to transform maintenance approaches, plant managers and other corporate leaders will need to remain flexible as the idea of smart buildings and factories continues to mature.
Solutions likely to deliver the best near-term gains will be those which can be retrofitted onto existing motors and other assets. As each asset comes online, the maintenance processes around it will shift from reactive break-fix solutions towards predictive, smart approaches that prioritize the right actions at the right time. This will allow maintenance personnel to put down the clipboards and focus on performing the tasks that improve machine health, reduce downtime and drive costs down across the organization.
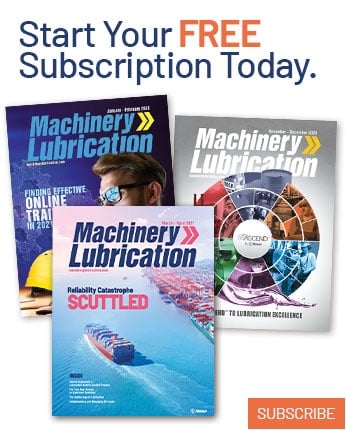