We’ve all heard from reliability experts that the “bathtub curve” – the poor, misunderstood bathtub curve – fails to accurately reflect a machine’s failure rate as a function of time. While there is much truth in the premise, there is more to the story, and gaining a true understanding about the relationship between failure rate and time can set you on your way to breakthrough reliability improvements.
Before we proceed, set in your mind the notion that the familiar bathtub curve is a conceptual model that generally defines all of the probable failure rate regions that a machine, component or individual failure mode might exhibit as a function of time, cycles or miles (we’ll stick with time for this article). These regions include infant mortality, constant failure rate and wear-out. Notably absent from the conventional bathtub curve is the linearly increasing failure rate scenario, which is commonly observed in equipment, but this can replace the flat area of the conventional curve.
Before reading on, please dispense with the notion that the curve is expected to illustrate the reliability life of your specific machines or systems over time.
To avoid a barrage of ugly e-mails, the assertion made by reliability experts that most machines exhibit a constant rate of failure as a function of time for most of their lives is generally accurate. The constant failure rate period often follows an infant mortality period (machine’s early life) during which the failure rate is elevated. Reliability-Centered Maintenance experts rightly utilize this information to modify and optimize maintenance plans. Again, for mechanical equipment, the failure rate often increases linearly as a function of time.
I want, though, to change your view of the constant failure rate period, the region in which most of your machines spend most of their lives once they survive infant mortality. This is often called the “random” failure period, which probably explains why it’s the least-understood region. While the failure rate may be mathematically random because machines fail to exhibit a definitive time relationship, that’s not to say the failures are without cause. Accepting that the failures are mathematically random can lull the individual or organization into accepting a belief that the failure rate can’t be controlled (a common misconception).
The typical and appropriate response to a constant failure rate is to develop an appropriate inspection and monitoring program and employ condition-based maintenance. Predictive CBM is still reactive; it’s a much more palatable form than waiting until the machine’s function is affected, but it’s reactive just the same. If we accept that the failure rate is random and fail to gain an understanding about why the failures are occurring, we miss opportunities to proactively alter the failure rate through changes in machine design, operational context and environmental condition control.
In reality, the constant failure rate period appears constant because: a) some of the failure modes are indeed random as a function of time, and b) there are so many unrelated failure modes contributing to the overall rate that the result appears to be random (Figure 1). For truly random failure modes, CBM is your best option. However, if the time to failure could be assessed individually on a mode-by-mode basis, you would likely find that many of the individual failure modes do indeed exhibit a time relationship – increasing or decreasing as a function of time. If a definitive relationship between failure rate and time for a specific failure mode can be established, you can take proactive measures to change the relationship. When all of the modes are lumped together to produce a constant failure rate, which creates a random appearance, all you can do is wait for the next failure, hope the monitoring program catches it and then react to it.
If you can establish a time dependency for a given failure mode that exhibits a clear central tendency (average) and a small amount of dispersion (standard deviation), and machine design, operating context or environmental context can’t be modified, you still have the option of selecting a “hard-time” maintenance task. I realize I’m running in the face of modern convention, which is oriented toward CBM as best practice. Despite the power of condition monitoring, hard-time scheduled maintenance tasks are still the easiest for which to plan and usually the least costly to execute. If a failure mode for a machine suggests a clear time dependency, and reliability objectives can be most effectively and efficiently achieved by addressing it using hard-time actions, then that should be your course of action. By all means, for failure modes with no clear time dependency, CBM is the preferred course.
Figure 1
In addition to providing the option to simplify maintenance with rationalized hard-time tasks, for failure modes that have a clearly defined time dependency, reliability engineers are armed with numerous opportunities to proactively improve reliability. Here are just a few:
1) Enable effective design changes. The design, build-up and commissioning phases of a machine’s life cycle determine its “genetic code,” or predisposition for reliability relative to operating and environmental contexts. By collecting failure data by individual failure mode, reliability engineers can more effectively support the design process. It’s one thing to tell design engineers that the machine ought to be more reliable (a typical scenario). It’s quite another to provide them with specific failure data broken down by failure mode. Armed with quality field data, design engineers can make specific changes. Without it, they’re left to guess.
2) Reduce early life failures. Machines are often plagued with costly early life failures following commissioning or major maintenance. By collecting and analyzing failure data by individual mode, reliability engineers can take specific actions to increase control over those factors known to result in early life failures, such as increasing precision during installation, creating and executing start-up procedures that reduce risk, etc.
3) Optimize condition monitoring intervals. Suppose a failure mode exhibits a time dependency, but it isn’t strong enough to warrant a hard-time maintenance activity. You elect to employ proven-effective condition monitoring tasks. Shouldn’t your knowledge about the failure mode’s time dependency influence your monitoring interval? Most condition monitoring routes are hard-time-based (monthly, quarterly, etc.). While the time dependency for a specific failure mode may not be strong enough to warrant a hard-time repair or replacement of the affected component or areas, it might warrant decreasing the monitoring or inspection interval as the machine enters the high-risk period.
To analyze failures by specific failure modes, you must become disciplined in the collection of field data, which will take work and diligence. Fortunately, you don’t need to reinvent the wheel. IEC Standard 300-3-2 (“Application guide – Collection of dependability data from the field”) provides a good recipe for creating a field data collection process. Likewise, IEC Standard 812 (“Procedure for failure mode and effects analysis [FMEA]”) provides a generic failure mode coding system so you can effectively categorize field data. It provides a good base. With some expansion, you can customize it to accurately reflect your machines and systems. Feed failure data into the FMEA. This provides the organizing structure for driving change.
So, let’s quit beating up on the bathtub curve and start putting it to work for us. While the bathtub curve may not define the reliability life of many plant systems, it provides a conceptual framework for understanding failure as a function of time. By breaking failures down on a mode-by-mode basis, it lets you break free of the random trap where you simply accept that random is uncontrollable.
Drew Troyer, CRE and CMRP, is the co-founder and senior vice president of global services operations for Noria Corporation. Since leaving Oklahoma State University, where he served as an instructor, his professional career has been devoted to improving machinery reliability. He served as product manager for Entek/Rockwell Automation and as the director of technical applications for Diagnetics Inc. His lengthy client list at Noria includes International Paper, Cargill, Goodyear, Texas Utilities, Reliant Energy, and Southern Companies. |
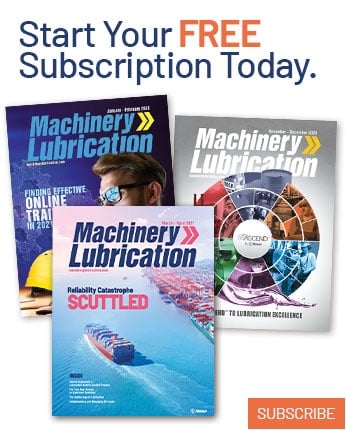