The operator performing an infrared inspection or thermographic survey collects a lot of valuable information while walking and observing. Some of these observations are collected using the powerful infrared imaging camera, while many others are simply things seen and noted by the person doing a survey. This article conveys practical ways to organize the findings into categories such as failure, operation and design. Within each category, the findings are each determined to be normal, low or high alert, and low or high fault. Faults are things that require further attention.
This is the third in a series of seven articles for Reliable Plant describing how the computer software inside today’s modern infrared cameras can do more than ever before to help the plant thermographer do his or her job far more efficiently and effectively. An automated thermographic inspection process resolves complex data into practical and understandable results or findings. A single infrared survey involves countless temperature measurements taken from thousands of components. The amount of data is immense. Without the automated thermographic inspection process, a successful inspection depends on human operators being vigilant and consistent in determining which temperature measurements are worth documenting and deciding how to report them. That process leaves room for inconsistency, missed findings and errors.
An application software routine should be used to help the thermographer resolve complex data into one of several dissimilar classifications. One approach is to classify all findings as “failure predictions,” “operational conditions” or “design characteristics.”
Fault lists may be created with each fault categorized under one or more of these classifications. For example, a relatively hot connection on a circuit breaker panel may indicate that a failure is in progress or may simply indicate that the power is flowing through that connection. If the connection is failing, the infrared inspection system can be used to monitor the progression of the failure over time and help if the condition is incipient or near late-stage catastrophic failure. It is appropriate to use multiple measurements over time to trend and thereby predict catastrophic failure. On the other hand, if the heat is simply due to power being turned on (e.g., normal operation), that fact is considered and may or may not need to be documented.
Fault-tree logic in on-board software can instruct the operator to further assess and document certain operational conditions to either verify normal operation or alert to unexpected operation. Anomalies in operating conditions are quite different from anomalies associated with failure in progress, and should be classified differently.
Sometimes the in-camera software is used to document observed design or quality factors. These factors are different from either failure predictions or operational conditions. For example, the insulation system in an exterior wall is supposed to have R-12 insulation. However, an infrared survey on a cold night outside the building may reveal far lower insulation is actually in place for one section of a wall. In this case, the specification or design or quality was below acceptable standard.
One step in the process of resolving complex data involves grouping faults or findings into three generally exclusive and dissimilar categories such as “failure,” “operational” and “design.” Another step is the assignment of alarm or severity level for each category.
Such characterizations are called grading levels, and typically this is done by assigning one of three or five different grading levels for each finding category. One implementation may describe three grading levels, like “normal,” “alert” and “fault.” Five grading levels may be described as “Normal,” “Low Alert,” “High Alert,” “Low Fault” and “High Fault.”
Human action or intervention is recommended when the condition reaches a particular level such as “Fault” on the three-level method or “High Fault” on the five-level method. Color indications are often used in association with alarm or severity status. Representations using grading levels and associated colors are shown in Table 1 and in figures 1 and 2.
Table 1. Grading levels and their significance.
Alarm or Severity |
Significance |
Color |
High Fault |
The problem is extreme |
Red |
Low Fault |
Problem identified needing human action to be taken |
Red |
High Alert |
Warning of impending action required |
Yellow |
Low Alert |
Differences are noticed |
Yellow |
Normal |
Nothing unusual to report |
Green |
It really helps the operator and the people seeing his or her reports if the reporting software is used to graphically represent the status values for failure, operation and design. This could be done different ways, such as in the form of a bar graph or multi-dimensional representation. The results may simply be portrayed as a numerical dataset. For example, the results could be (1,4), (2,0), (3,2), where the first number in the set represents the category and the second number represents the grading level.
A multi-vector graphical representation may be used including two or three or more dimensions. For example, in a two-vector representation, one category may be represented on the X-axis and a second category on the Y-axis of an X-Y graph. The severity level may be shown as increasing numerical values starting at the intersection (origin) with levels of alarm or severity increasing as the distance from the origin increases. A tri-vector representation adds a third dimension to the graphical representation using a radar-type plot. An example of a tri-vector graphical representation is shown in Figure 1.
It is possible and practical to integrate findings from alternate sources in addition to infrared inspection information into a multi-category format. For example, one may record observations including process variables such as temperature, pressure and flow conditions while accomplishing infrared surveys. These operational conditions can contribute to the overall findings. The same can be applied to information or data gained from associated condition monitoring information such as vibration analysis or ultrasonic analysis or oil analysis information. All of these can be interpreted in a structure like that shown in the Table 1. Those findings can be accumulated along with infrared observations during an infrared survey.
Generally, all of the findings associated with each classification category are considered in the assignment of an overall severity level for each category, in other words for “failure,” “operation” and “design.” Normally, when multiple parameters or anomalies in one category are considered, the highest one determines the overall level. However, to avoid nuisance alarming, false negatives and false positives, consideration may be given to multiple measurements, data and information from various sources when determining the overall values for each of these three categories.
By resolving the complex data from infrared surveys into these three dimensions (categories) and assigning a grading level to each, anyone who reads the report can easily interpret the significance of the findings.
A huge advantage for this approach to thermographic inspection is an automated reporting process that is far beyond what could be done earlier. Adobe PDF or Microsoft Word or other type reports may be fully formatted in the portable IR system at the completion of the area survey. Two examples of one page of a report are provided in figures 1 and 2.
This article is based on things first disclosed in Garvey, et. al., U.S. Patent Application SN 10/872,041, "Method of Automating a Thermographic Inspection Process."
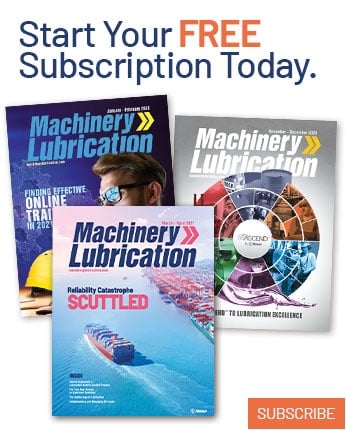