
Auto manufacturers today are building multiple models on the same line, which presents the challenge of unique part numbers within a plant exploding to unprecedented levels. The only way to manage this increase is with accurate and quantifiable data.
Even with good data collection, with so much of it, effective data management becomes absolutely critical. Insights into data collection and analysis can ultimately help optimize market competitiveness. Here’s how.
Define Current State, Identify Missing Data and Incorporate Informed Assumptions
Before a project team can manage its data well, they must first define the current state, identify what data is missing or suspect, and what is relevant to the problem at hand. Making informed hypotheses and educated assumptions based on experience as well as asking the right questions at the outset can help refine those assumptions as more data comes in. A project may start 18-24 months in advance of a new program launch. At that point, details can be scarce, but surrogate data — perhaps from a previous program — can provide enough insight to make initially sound assumptions, which will ultimately be replaced with real data.
The accuracy of early data is improved gradually through change management techniques as updates are added to the data management tool. There can be thousands of changes in data from the start of the project to the launch, and proper adjustments and analysis during the change management process can save time and effort. Changes also come with more frequency the closer the project gets to launch, so the team should be prepared to handle an increase in change requests during the later phases of the project, particularly when information about suppliers becomes available.
For example, where suppliers are located can help determine how much space is needed for inventory. If materials come from overseas, there may be a need for an additional significant timeframe of inventory to be on hand.
Ultimately, all of this data leads to a plan for every part (PFEP) – from the part number to where it is sourced, the packaging information, who will deliver it, how often it will be received, and where it is received at the plant. Once at the plant, consideration of each part continues from where it will be delivered, how it will be delivered, the method with which it will be displayed on the line, and how empties will be moved out of the facility into the supply chain. Going forward, it is important for the project team to take ownership of the change management in order for the PFEP to be accurate.
Collect and Vet Data with Insight
Data does not have to be perfectly accurate early on. Priorities should center on organizing data so it is easy to manage and adjust to change. Learning how to identify the point of diminishing return, which typically first shows up on container turns, is important. Some containers don’t turn very often, while others turn multiple times in an hour. Understanding which are critical will help create appropriate priorities.
In general, the number of permutations and combinations can often be represented by the 80/20 rule in many manufacturing industries. Typically, if a part has 15 different permutations, 20 percent of all those parts might represent 80 percent of the usage. The other 12 will represent the remaining 20 percent. In the automotive industry, it is not unusual for a main body wiring harness (MBWH) to have more than 100 part numbers, many of which will not have significant usage. The 80/20 rule resonates with the cost of carrying that complexity and understanding usage of both low and high runners.
Vetting the data once it has been collected requires a familiarity in terms of knowing whether a part is a small lot part or a bulk part. In addition, you should know whether the part is going to be sequenced, kitted, cross-docked or direct-delivered in bulk or on a small lot route, often referred to as a “milk run.” Project team leaders start with a list of the assumptions, which is shared with the team for feedback. This includes assumptions about sourcing, how many days of inventory on hand, and methods of delivery — small hand-carried totes, full pallets, layered pallets or mixed pallets. After the team weighs in, the assumptions are documented and used as the project progresses until more concrete data confirms the assumptions or prompts a change.
Use the Right Tools for the Job
Utilizing a well-developed data management tool can help optimize the data used for the design and determine how much space, labor and equipment are ultimately required. A lean material planning tool ties together dock analysis, material flow analysis, storage analysis and empty container analysis, working out of a consistent and integrated data set. It is important to have one repository for all data so that nothing is overlooked while updating data in multiple tools.
These types of tools can optimize storage by managing and maintaining a number of factors, including whether on the floor or in racking, how deep the lanes are, and the aisle widths required for material handling. They can also assist with space-planning directives, such as flow-through or back-to-back storage, whether there is one-way or two-way traffic, and ensuring management of first-in/first-out (FIFO) practices. For example, if base constraints will not allow for flow-through storage, it may be necessary to have multiple lanes of storage for each part number so FIFO can be managed throughout the process. If space is at a premium, a pallet rack may be a better choice for low-runner parts. The right tools will help optimize space through the design process without ignoring other important factors.
Early in the planning process, the proper tools can provide data at a block form level for layouts. More detail will be added as the plan progresses, such as footprints within the lanes. For example, the layout may use a 48-inch lane for pallets that are 42 or 45 inches wide. While this may seem to leave unused space, it provides necessary flexibility. Eventually, the plan will include details on exactly how much space is used, how many stacks there are and how many containers are in each stack, with an address for every part.
By using suitable tools, designers can work within the existing constraints of the facility, such as columns, restrooms, offices, “monuments” or charging areas for equipment. The layout of the plant also factors into the material delivery strategy — whether parts flow through the facility or enter and exit at the same docks. Achieving the most efficient process flow will depend on how many docks the facility has, their location and whether there is an offsite warehouse feeding the production line. Appropriate tools can monitor all of these conditions and data during the design process.
Visualize Material Storage
The details of the material storage solution drive the layout design work. It is possible to estimate space requirements through calculations, but ultimately a layout is needed to provide a more accurate visualization. Until the facility is laid out with aisles, working around the existing columns and other internal constraints, it is often hard to know what the actual space needs will be.
With a layout model, different scenarios for storage and movement can be tested to avoid pitfalls and to ensure a more optimal solution. Storage requirements can be put into a 2-D or 3-D environment to enhance visualization.
Integrate Storage and Flow Analysis
Efficient storage is one part of the design, but the flow of material to the production line is even more critical. The highest running material should be laid out so that it has the shortest path to the point of use. If an offsite warehouse is used, the travel distance from the dock to the market to the line, as well as efficient removal of empty containers, must be part of the plan. The end result of the material flow analysis quantifies the material handling labor and equipment that will be needed to execute the process.
Material flow and storage design work in tandem, so each should be considered when planning the other. To balance cost, quality, delivery and safety without compromising any of them, robust tools will be needed to quickly evaluate the space and provide data-driven solutions that will work in practice.
Optimizing Market Competitiveness
Because planning must be done before the line is up and running, it is essential to have a data-driven solution. Good data allows the project team and stakeholders to confidently say “I know” instead of “I think” when planning the details of the process.
As in most business plans, the highest value to the customer is always paramount. This process is geared toward reducing waste and maximizing efficiency, thereby optimizing market competitiveness. Ultimately, the key to achieving these interrelated goals is in the data.
About the Author
William Herrmann is the vice president of productivity solutions at Ghafari Associates. He can be reached at wherrmann@ghafari.com or 313-425-3665.
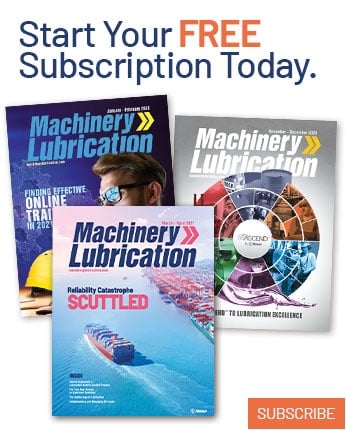