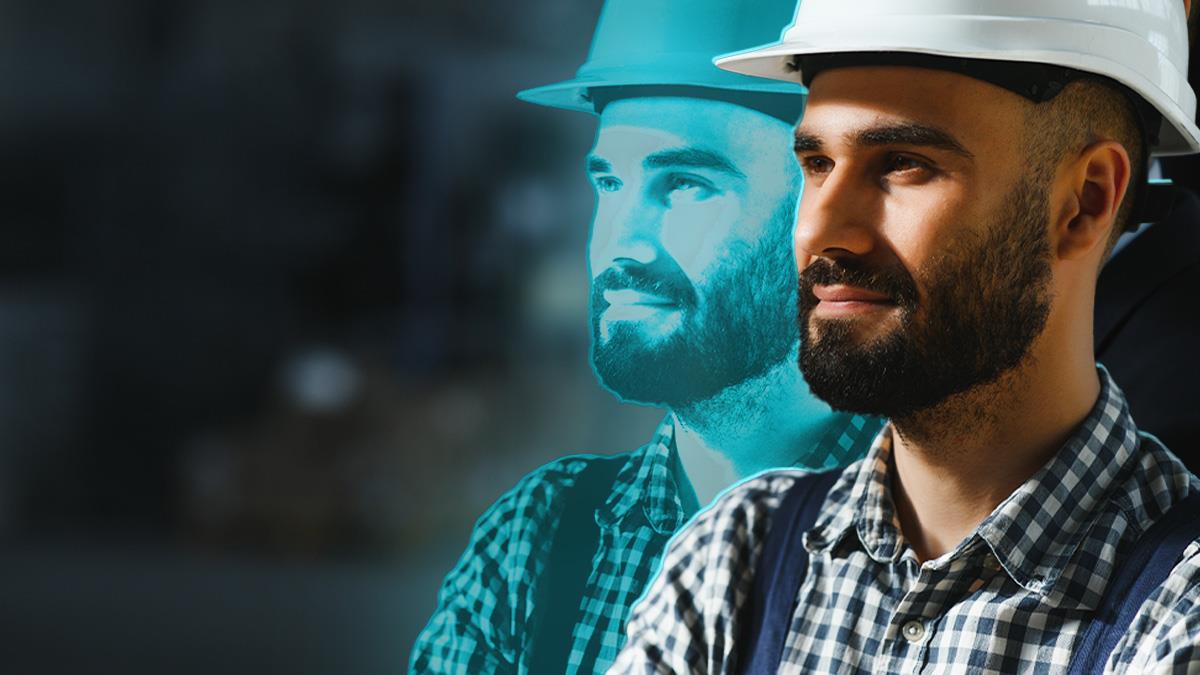
The aftermath of COVID-19 has reshaped the landscape of asset management, particularly in heavy industries like refineries and manufacturing plants. With this challenge comes an opportunity to drive a paradigm shift regarding how plant operations are managed.
Industrial facility owners and operators are now grappling with several challenges, like global supply chain disruptions that may impact “run and maintain” operations, while striving to meet growing production demands. What keeps them up at night are the risks they cannot see, particularly when it comes to unexpected asset issues and possible long-term equipment damage.

To help manage the lifecycles of all assets in an industrial facility, organizations are looking for innovative solutions to optimize their equipment performance while ensuring safety and operational reliability. This includes data integration and standardization and adopting digital twin technology that provides predictive asset maintenance analytics.
What is Digital Twin Technology?
Today, engineering and design teams use dynamic process simulation models to view the current state of plant operations. This allows them to quickly
pinpoint operational bottlenecks, unit shortcomings, design limitations, and the adequacy of relief systems. However, it takes advanced machine learning (ML) capabilities to model and predict the future state of plant operations.
Digital twin technology leverages machine learning algorithms to aggregate, integrate, analyze, and compare historical operational and design data against current data to form the statistical model. The result is a virtual clone of each unit in a facility, replicating its physical counterpart’s functions, features, and behaviors, along with the mechanical and electrical systems feeding into it.
Digital twin models also include a facility or industrial site's physical infrastructure and intricate web of plant processes, including procurement and supply chain logistics, to create a digital operational environment.

Example of Digital Twin Technology Virtual Clone. Source: Anvil Corporation
Receiving the right information at the right time prevents equipment failures. Digital twin’s advanced machine learning capability provides owners/operators with predictive analytics based on patterns and correlations in the data.
Armed with this information, owners/operators can see:
-
How each unit may age and change over time
-
When a unit may experience failures
-
When to order long lead items based on procurement/supply chain system models
-
When to address an issue before it occurs
A question that frequently arises relates to the difference between artificial intelligence (AI) and machine learning, as both advanced technologies deliver predictive analytics. The difference is:
-
Machine learning provides owners/operators with objective information on which to base their decisions
-
AI provides a subjective approach to decision-making by recommending courses of action.
The intent of digital twin machine learning is not to replace human interaction and evaluation but to make the interaction non-intrusive and ever-learning.
In addition, AI is an open-source technology operating across the Internet, which can potentially violate proprietary information, whereas digital twin’s machine learning software is confined to a particular company, facility, or location.
By simulating future operational scenarios with digital twin models and
machine learning predictive analytics, owners/operators can optimize their resources, processes, and maintenance schedules, strategies, and setups.
Digital twin technology also helps owners/operators predict when to schedule a turnaround and when to order long lead items before a shutdown. This is especially critical for remote sites with longer lead times for equipment, giving owners/operators the peace of mind of knowing that they will have their procured equipment onsite when needed.
Building a Digital Twin Blueprint
The first step to building a digital twin blueprint is to locate and feed all historical data, including inspection records, new codes, re-rates, and current operational data, into the digital twin software to develop a virtual and reusable prototype of each physical asset in a production facility.

Example of Digital Twin Technology Virtual Clone. Source: Anvil Corporation
A key part of this step is ensuring that correct monitoring instrumentation is in place to feed the digital model constantly. Both historical and original manufacturing guidelines can be programmed as “alarm” points in the model to alert owners of potential upsets or failures.
Digital twin machine learning’s function takes it from there, aggregating, cross-referencing, and instantly integrating all disparate data from multiple sources in real-time. By comparison, it would take an engineer weeks to calculate the same information manually.
Once all the data has been collected and integrated, machine learning compares current performance against what the unit was initially designed for to instantly flag abnormalities or deviations from expected behavior. This helps engineers instantly identify an area of concern to be investigated further and virtually validate and scale the models to expand their capabilities without disrupting facility operations.
Digital twin models then predict what lies ahead by simulating future operational scenarios to determine how units will evolve over time and when unit components will need to be replaced.
As digital twin technology continues to evolve, other types of physical evaluations may become obsolete, such as on-line test runs. For example, these advanced models can predict how systems react to process variable changes without upsetting a facility’s day-to-day operations, eliminating off-spec products and potential safety concerns associated with real-world test runs.
Challenges of Digital Twin Technology
Output is Only as Good as Input
The leading challenge of building digital twin models is not so much the technology – it’s determining if the aggregated data from several sources based on multiple variables feeding into the models is based on reliable information.
For example, how does an operator know when a part or system is already in a current state of failure when building the models?
This involves
defining reliable information. Reliable information typically comes from more data that digital twin’s machine learning has cross-referenced and validated—data from process and equipment monitoring instrumentation integrated into a smart, automated plant network.
For example, capturing one set of data on a unit's performance is not enough. For more reliable information, it is important to capture data on the unit’s supporting infrastructure as well. It could be that piping into the unit or electrical components powering the unit are already in a state of failure, impacting multiple other units operating with the same mechanical and electrical parts and systems.
The Power of Data
More data gives owners/operators more realistic views of current operations, which helps to build a more accurate digital twin model. With integrated and validated data from multiple inputs, digital twin models can:
With digital twin models, facility owners and operators can run and maintain their operations during turnarounds and peak production seasons.
However, it is still critical for an engineer to fill in the subjective operating data. Metallurgical considerations, along with the chemical and physical effects of process streams on equipment and piping, will still require engineering knowledge and expertise to fully use digital twin information.
The fact that millions of parts make up hundreds of systems in any industrial facility underscores the criticality of keeping accurate maintenance records; a digital twinning model’s output is only as good as its input. Therefore, garnering stakeholders' willingness to adopt the technology depends on their degree of trust and whether they believe the information is accurate or not.
Trust in the Technology
Implementing digital twin technology successfully requires access to the right software tools and trained specialists. Process simulation software companies are now purchasing interface technologies to better develop digital twin models.
The challenge lies in ensuring that the interfaces are correctly integrated to produce an accurate baseline of information. It takes trained specialists knowledgeable about digital twinning technology and processes
using industry metadata to ensure that all the historical information feeding into the models is correct.
Summary
Digital twin’s advanced machine learning algorithms, predictive analytics, and modeling capabilities offer a new cost-effective asset management option for facilities of all sizes. By working with engineering consultants and using the right software, facility owners/operators can quickly implement the technology and integrate it into their operations.
The technology also produces multiple cost benefits, including:
-
Operations and energy efficiency
-
Improved reliability, safety, and maintenance forecasting
-
Reduced risk, downtime, and unnecessary maintenance costs
Embracing digital twin technology will be essential to staying competitive in rapidly evolving industrial markets.